The YOLO (You Only Look Once) series has solidified its reputation as a top choice for object detection, acclaimed for its remarkable speed and precision. With each successive version, the YOLO family consistently advances the field of computer vision, and YOLOv10 is no different in pushing these boundaries.
In this guide, we'll walk you through the steps to train a YOLOv10 model with a custom dataset. We'll use an example of training a vision model to identify chess pieces on a board. However, the principles outlined in this guide are flexible and can be adapted to any dataset you choose.
What is YOLOv10?
Released in May 2024, only three months after YOLOv9, YOLOv10 is the latest iteration of the YOLO series, continuing its legacy while introducing significant innovations that set new benchmarks in object detection capabilities.
YOLOv10 builds upon the advancements made by YOLOv9 and introduces several key enhancements. Notably, YOLOv10 eliminates the need for non-maximum suppression (NMS) during inference, which reduces latency and enhances efficiency. This is achieved through a consistent dual assignment strategy that improves the training process by providing rich supervisory signals and aligning the training and inference stages more effectively.
Performance and Efficiency Improvements in YOLOv10
The YOLOv10 model is available in six variants, categorized based on their parameter count:
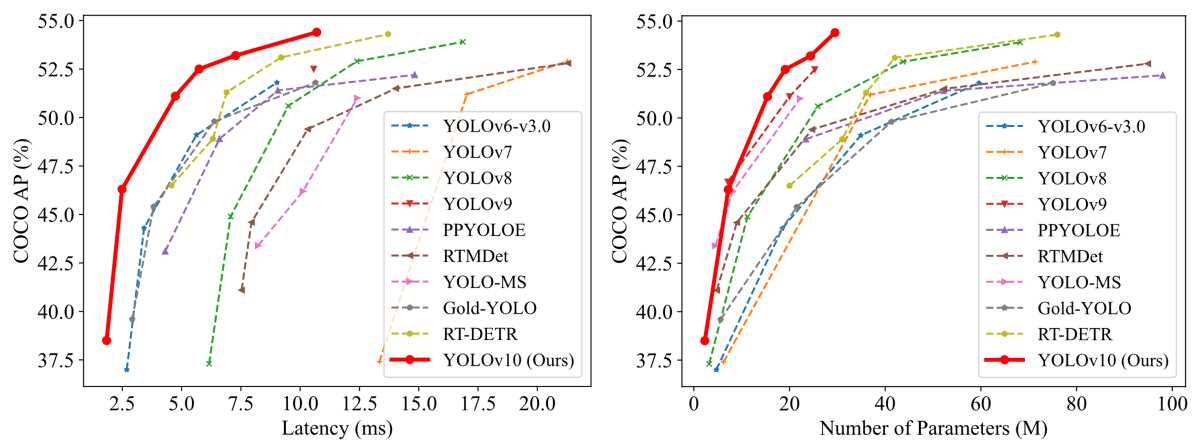
Compared to YOLOv9-C, YOLOv10-B achieves a 46% reduction in latency while maintaining the same performance level. Additionally, YOLOv10 showcases highly efficient parameter utilization. For instance, YOLOv10-L and YOLOv10-X surpass YOLOv8-L and YOLOv8-X by 0.3 and 0.5 average precision (AP) points, respectively, while using 1.8× and 2.3× fewer parameters. Similarly, YOLOv10-M matches the average precision of YOLOv9-M, but with 23% and 31% fewer parameters, respectively.
Architecture and Innovations
YOLOv10 introduces several architectural innovations aimed at enhancing both efficiency and accuracy in real-time object detection. The architecture builds on previous YOLO models, integrating new design strategies to improve performance.
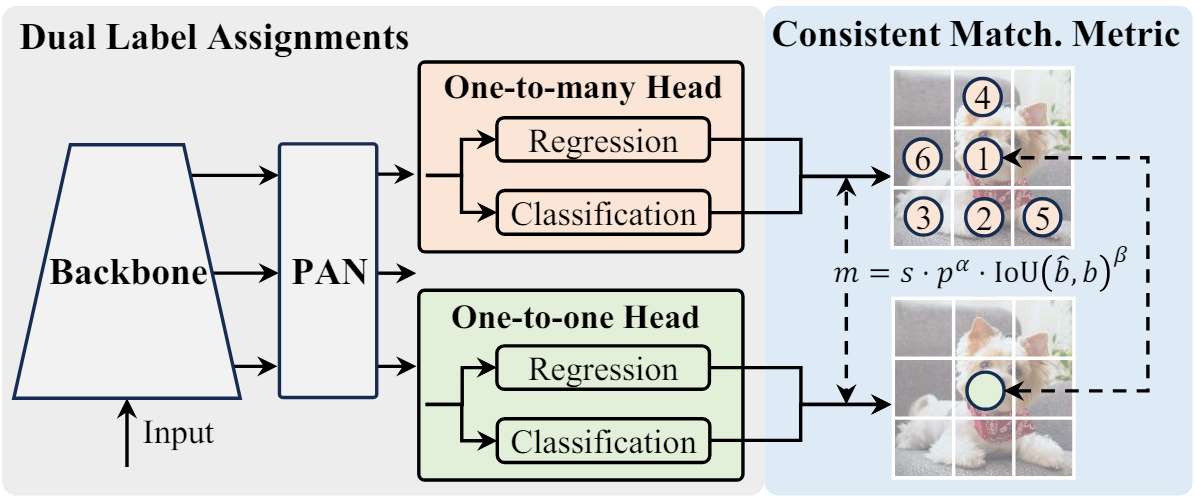
Key Components
1. Backbone:
2. Neck:
3. Head:
Innovations
1. NMS-Free Training:
2. Holistic Model Design:
Comprehensive optimization of model components from both efficiency and accuracy perspectives includes:
3. Enhanced Feature Extraction:
Easily train YOLOv10 on a custom dataset
The Ikomia API enables efficient training and inference of the YOLOv10 object detector with minimal coding effort.
Setup
To begin, it's important to first install the API in a virtual environment [3]. This setup ensures a smooth and efficient start to using the API's capabilities.
Dataset
For this tutorial, we're using a Chess Pieces dataset from Roboflow, which includes 693 images [4]. This dataset is ideal for training our custom YOLOv10 object detection model. It contains 12 labels: pawn, knight, bishop, rook, queen, and king, each in both black and white.
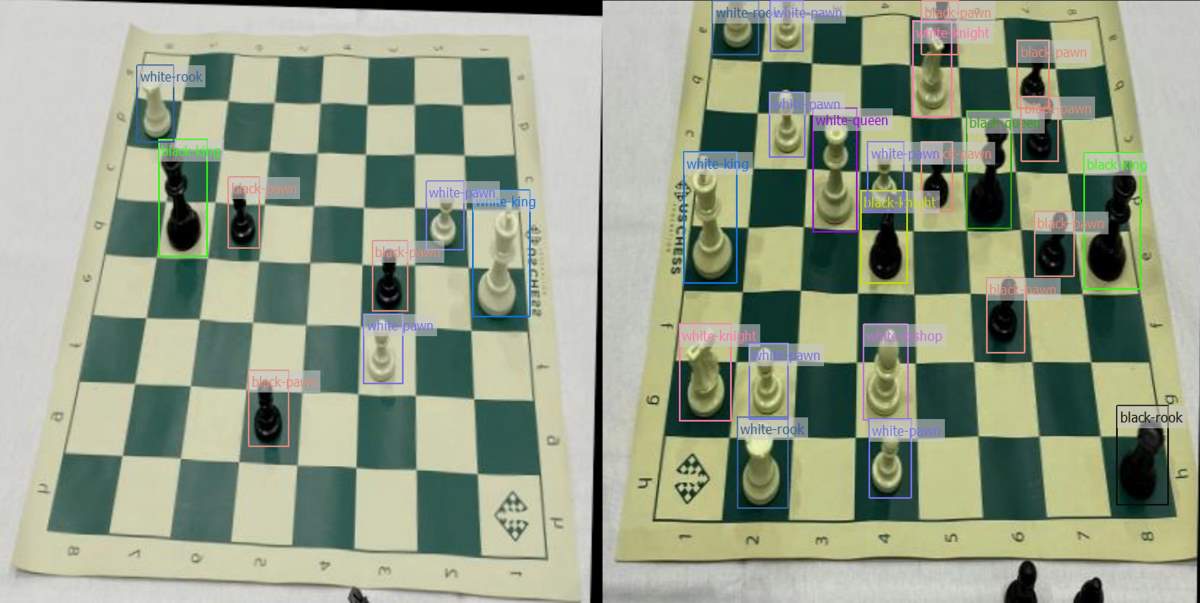
Train YOLOv10 with a few lines of code
You can also directly charge the notebook we have prepared.
Here are the configurable parameters and their respective descriptions:
The training process for 50 epochs was completed in approximately 30mins using an NVIDIA L4 24GB GPU.
Performance of our custom YOLOv10 model
Once your model has done training, you can assess the performance by looking the graphs produced by the YOLOv10 training process. These visualizations represent various metrics that are crucial for understanding the effectiveness of your object detection model.
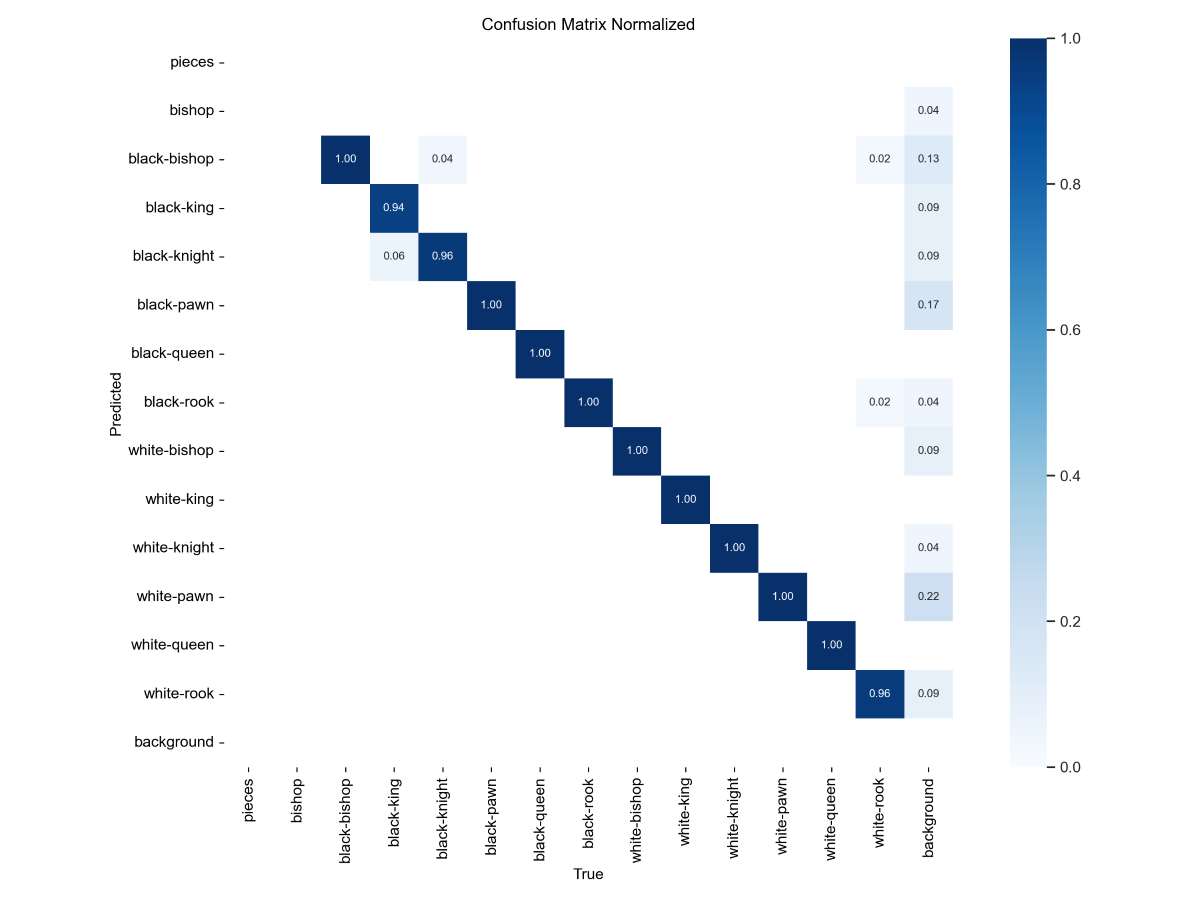
The confusion matrix indicates that the model shows high precision for most classes, such as black-pawn, black-rook, and white-king.
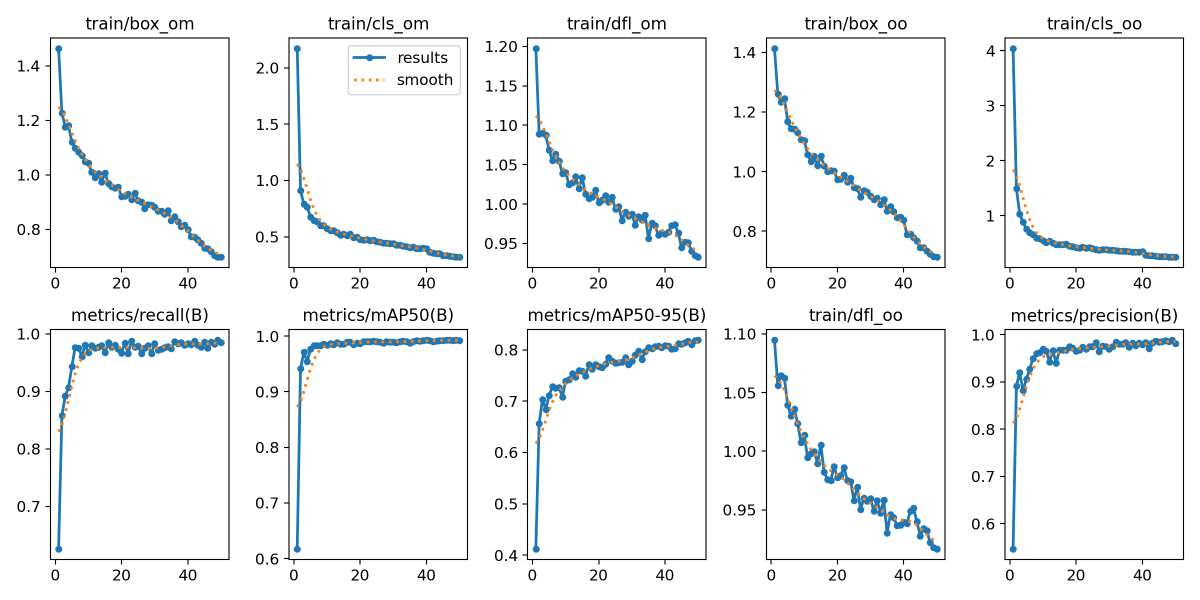
Looking at the box and classification Losses, both types of losses steadily decrease, indicating improved accuracy in object localization and classification over time.
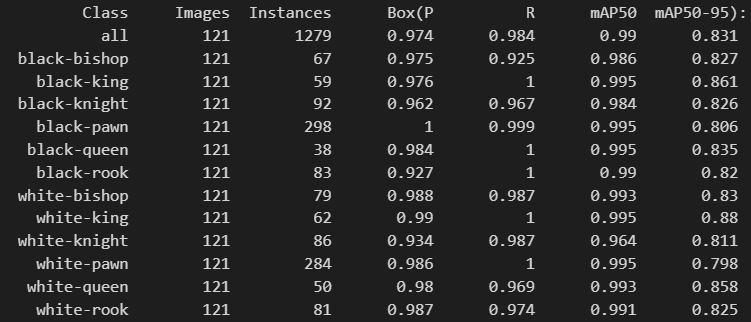
For the performance metrics, the recall and mAP show a consistent increase, demonstrating the model's enhanced ability to detect and accurately classify objects.
Overall, the model has learned effectively, showing high precision, recall, and mAP values. However, based on the loses curves we can see that the model did not have enough opportunity to converge. Extending the training period could further improve performance by reducing minor classification errors and enhancing detection accuracy.
Run your fine-tuned YOLOv10 model
We can test our custom model using the ‘infer_yolo_v10’ algorithm. While by defaults the algorithm uses the COCO pre-trained YOLOv10m model, we can apply our fine-tuned model by specifying the 'model_weight_file' parameters accordingly.
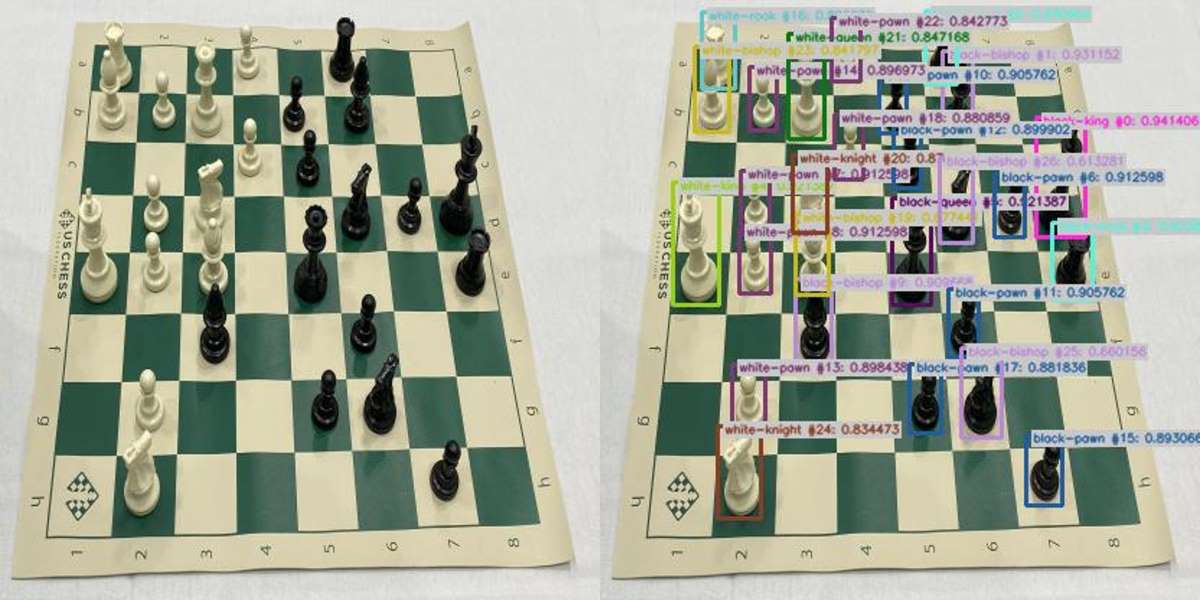
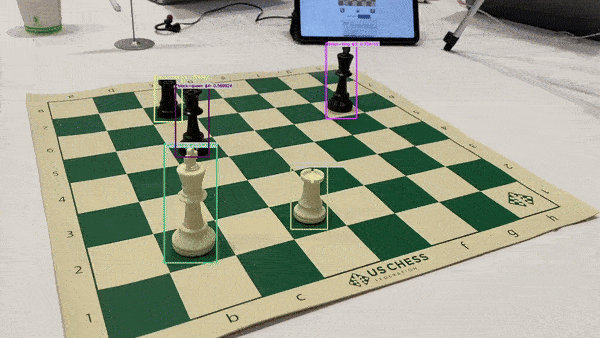
Our model successfully identified all the chess pieces, a first step towards developing a robot capable of beating Magnus Carlsen 😄! This achievement highlights the potential of the YOLOv10 model in accurately detecting and classifying complex objects.
We demonstrated how to train the highly performant YOLOv10 model on a custom dataset. The process outlined in this tutorial is easily adaptable to any dataset, making it a versatile tool for various applications. By leveraging the Ikomia API, this methodology can be seamlessly integrated into your projects, allowing you to harness the power of YOLOv10 for efficient and precise object detection tasks.
With further training and optimization, this approach can be extended to a wide range of real-world scenarios, pushing the boundaries of what's possible with AI-driven object detection.
0 Comments